Embeddings is the knowledge that exists in the “brain” of an LLM system. What an embedding does is simple: It gets as input a text, and it creates a vector for this text. E.g, it gets the text “Hi, my name is Fotis” and it creates a vector [0.2, 0.1, ….-0.3]. Then, it stores all…
How to Build a Motivating and Fair Sales Compensation Plan in your Startup
As a startup, you’re facing aggressive sales targets, and it might be a challenge to structure a fair bonus incentive scheme for your sellers. Let’s explore an approach that could work well for you. TLDR Mindmap: Here is a mindmap of this blogpost, created by ChatGPT+Whimsical: First, let’s consider the most common challenges from a…
OpenAI + HR Platforms: 11 ideas to integrate OpenAI & ChatGPT in your SaaS HR Platform
Integrating OpenAI into your SaaS platform can bring a wealth of benefits for your customers. Imagine automating repetitive tasks, improving decision-making, and even forecasting talent needs. In this article, inspired by a discussion with a Polish HR-Platform SaaS vendor, we’ll dive into how OpenAI can be leveraged by software development companies, startups, scaleups, SaaS vendors…
Unlocking the Potential of OpenAI and ChatGPT on Azure: A Guide for Startups and Digital Natives Companies
OpenAI and ChatGPT are the new cool kids on the block, so let’s have a look how startups and software development companies can leverage them to create cutting-edge applications. OpenAI is already available as a managed service on Azure and ChatGPT is coming soon. In this post we’ll have a look at: What is OpenAI…
Innovation in Retail from China and around the Globe
Retail is an amazing area for tech innovation: AI, Big Data, IoT, Drones, Autonomous Delivery Vehicles, Social Media, Influencers…It’s all there. This week we had a very interesting retail industry session on the latest trends and innovations by Noah Herschman, our Online and Offline Retail Innovation Leader, and as we are working with many ISV…
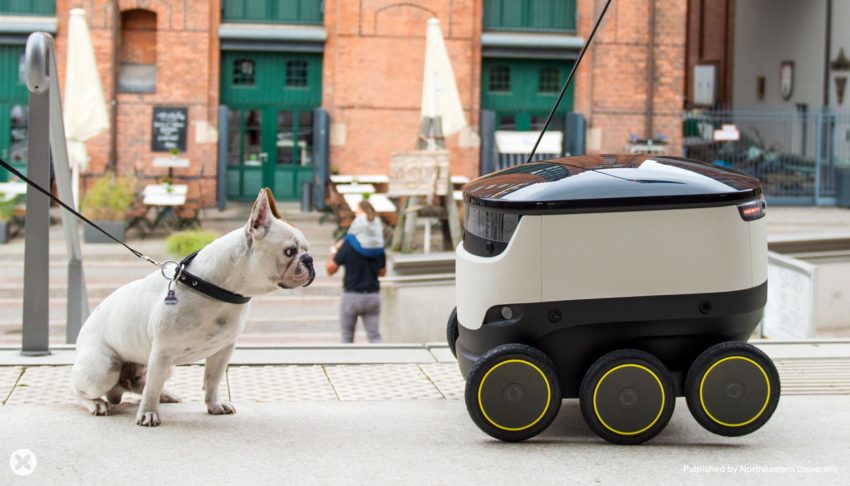
Service as a Software Business Model
Service as a Software business model is a new trend that emerges thanks to the advances of AI and Machine learning. Here are some thoughts on what opportunities this might bring in the next years.
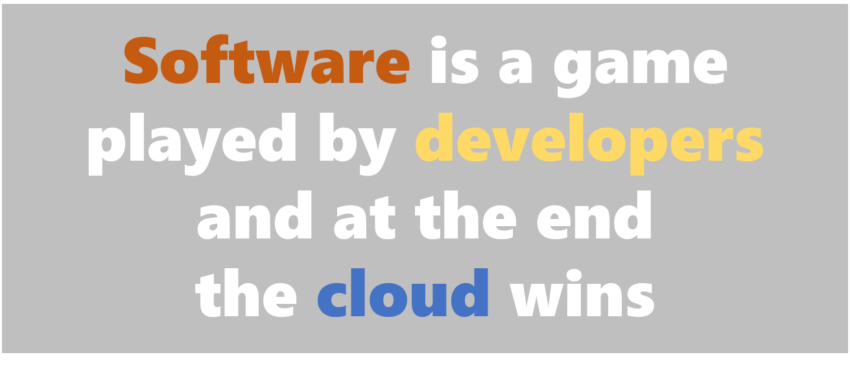
12 Reasons to Move your Software Solution to the cloud
In my discussions with software houses that already have an on-premise software solution, the question of “Why should we move to the cloud?”, often comes up. I know it is 2019 and in many parts of the world the discussion is not “Why Cloud?” but “Which Cloud?“, but a lot of software companies founded before…
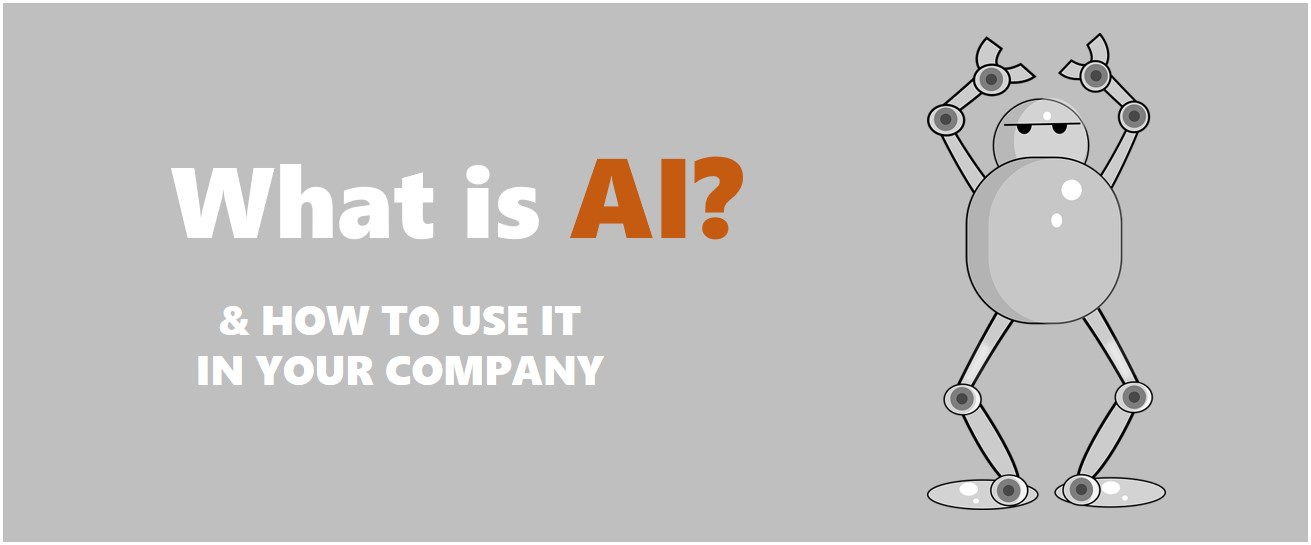
What is AI (for non-technical people) and how to use it in your company
Below you can find my notes from a very interesting online course on AI, from Andrew NG, an AI team leader at some of the most prominent AI projects in the world, which is available here. So, let’s have a look at what AI is all about and what AI can do for your company….
Is it possible to startup if I already work as an employee?
Often, the startup ideas come from employees that see a gap in the market they are working with. E.g., an employee at a corporation who finds an opportunity to create a mobile app in the tourism area and gets on board a couple of team members to work together on the idea, on a part-time…
What is a fair share of equity that each startup member should get?
A common question between co-founders when they start a new venture, is how to split their shares in the startup. Let’s suppose that you have an idea for a startup and you decide to co-fund your startup with another two persons. So, you are three co-founders. Should you split equally the equity in your startup…
How to Pitch Your Idea and Your Startup
At the Founders Institute in Athens, we are working with teams on pitching their ideas and try to develop them into viable startups. The teams get better and better as the number of pitches increases and the feedback they get gets more concrete, so here is a guide on how you can get a…
30 Startup Buzzwords You Should Know
There is a specific language that is used in the startup world. Yes, it contains many buzzwords and there are also humorous infographics on them, while many people -who are many years in the space- make fun of new teams over(ab)using them (read posts at quora). However, you should still invest some time in learning…
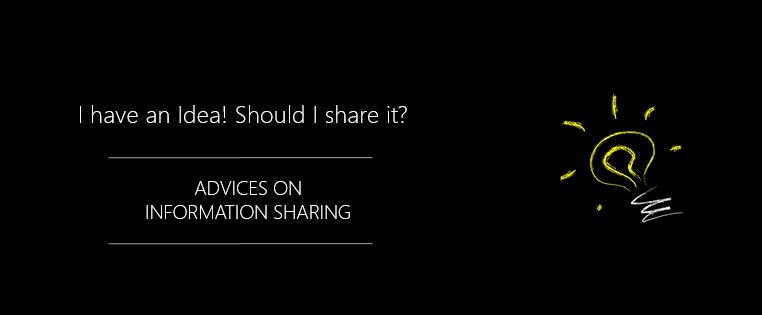
I have a startup idea! Should I tell it to others?
Yesterday we had a Startup Pitch presentation at Founders Institute in Athens (click) and one of the questions that came up was: “I have an idea for a startup. I am afraid that if I tell it to others, someone may steal it. Should I tell my idea and how can I protect it?”. The…
A Quick Guide to Remarketing
Remarketing (or retargeting) is a relatively new trend in online marketing, so here is some information on how you can use it to increase your customer’s loyalty, conversions and sales.
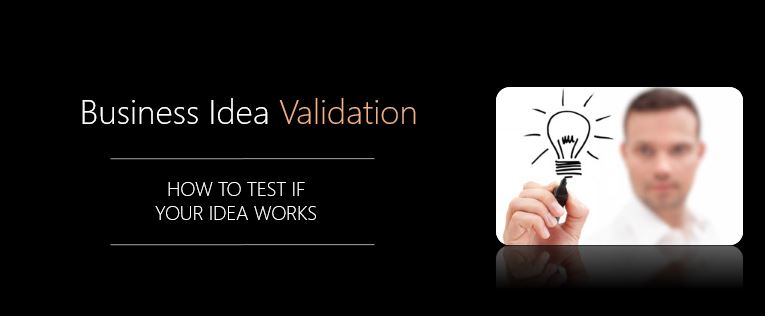
Can your startup idea become a hit? How to validate your business idea.
You have an idea for a new business startup. And before you jump in writing code, developing it or pouring money and effort into it, you want to validate if this could work at your targeted customer audience. Here is a methodology on how you could validate your business idea:
Mobile App Economy: 10 Questions Answered by Vision Mobile
A lot of Greek Startup teams focus on developing applications for mobile platforms, so we asked the opinion of the experts :). This is a guest blog post from Andreas Pappas (@PappasAndreas), Senior Analyst at VisionMobile (visit VM’s great blog here).
How to Build Trust for your Startup
We had the TIE Athens Bootcamp yesterday and one of the subject that was discussed is: I am startup, my brand is not that well-known, so how can I make others (website visitors/investors/potential customers) trust me? Here are some ideas on how you can build trust for your startup:
How to Name Your Startup,Mobile App & Software Product
Finding how to name your startup is one of the first problems you will need to solve. While some argue that you should leave the struggling to find a name for a later stage and focus at first to just build your product, it is still a nice investment of time to spend some days figuring out…
Business Plan and Business Model for Startups
Here is the problem: You are a team of great developers and you are building your dream product. But you have no marketing or business skills. And you’re still thinking of getting some 50k accelerator/seed funding, or even a bigger VC investment.
Athens Startup Weekend 2013: How to make the most out of it
In a few days the 5th Athens Startup Weekend takes place. ASW is a 54 hour event which can help you to get your business idea to the next step. At ASW you can pitch your idea, get developers/designers/business people in your team, get support from advisors and work intensively on your idea for 54 hours. At…
3d Printing and Business Opportunities
3d printing is the next trillion dollar opportunity according to many economists. This is a post to raise awareness to the greek startup ecosystem and examine some business opportunities.
Startup Events,Accelerators,Incubators & VCs in Greece
Do you have an idea and you want to build your own startup? Or you already have your own startup and want to take it to the next level? Here is a list of resources that can help you:
The "Funded" | Greek Startups with Seed/VC Investments
This is the list of some Greek startups that have been funded (seed or VC level) in the last months, according to online published information. Last updated on 15 July 2016
TechCrunch Athens | Startups Meet up | Jan.13
A few days ago the Techcrunch Meetup Athens event took place in the Museum of Benaki (#tcathens or #tcath, fotos from vrypan here), with more than 400 attendees from the startup community of Greece. The event was organized by Niko Bonatso and Alexia Tsotsis, with the support of OpenCoffee and Microsoft Innovation Center in Athens among others and…
Ψάχνετε χώρο στη Θεσσαλονίκη για να ξεκινήσετε να αναπτύσσετε την ιδέα σας? Γνωρίστε το Thermi-Link
Την Παρασκευή είχα την χαρά να συμμετέχω ως ομιλητής στην προσυνεδριακή εκδήλωση του VC Forum 2011 (http://11.vcforum.gr/11/pressroom) , στην οποία έγιναν και τα εγκαίνια του Thermi – Link (http://www.thermi-link.com/). Tι είναι το Thermi – Link και πώς μπορεί να σας βοηθήσει? Είναι ένας χώρος co-working – σκεφτείτε κάτι αντίστοιχο του Colab που υπάρχει στην Αθήνα…